As a development team, our goal is to deliver software that solves business problems. The business’ goal is to provide value to the customers as quickly as possible. “Monolith to microservices” is not a business goal. Business stakeholders don'…
Are you ready for microservices? Pt 1: Need, alternatives, and ability
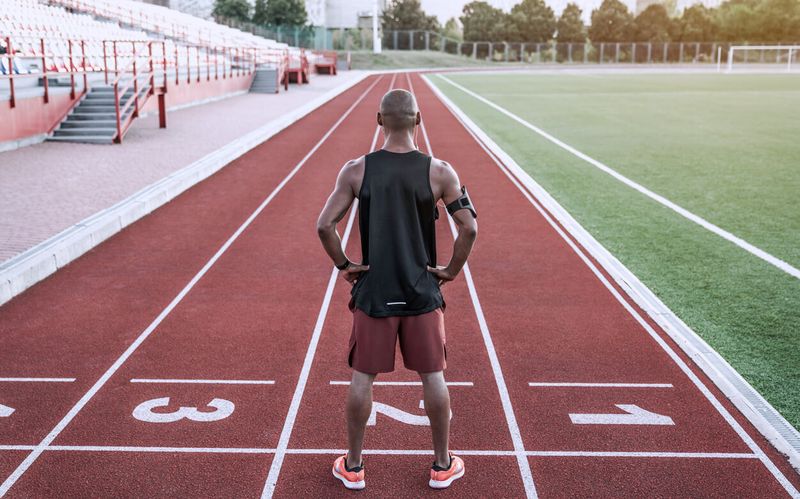